The Importance of Data Labeling in Machine Learning
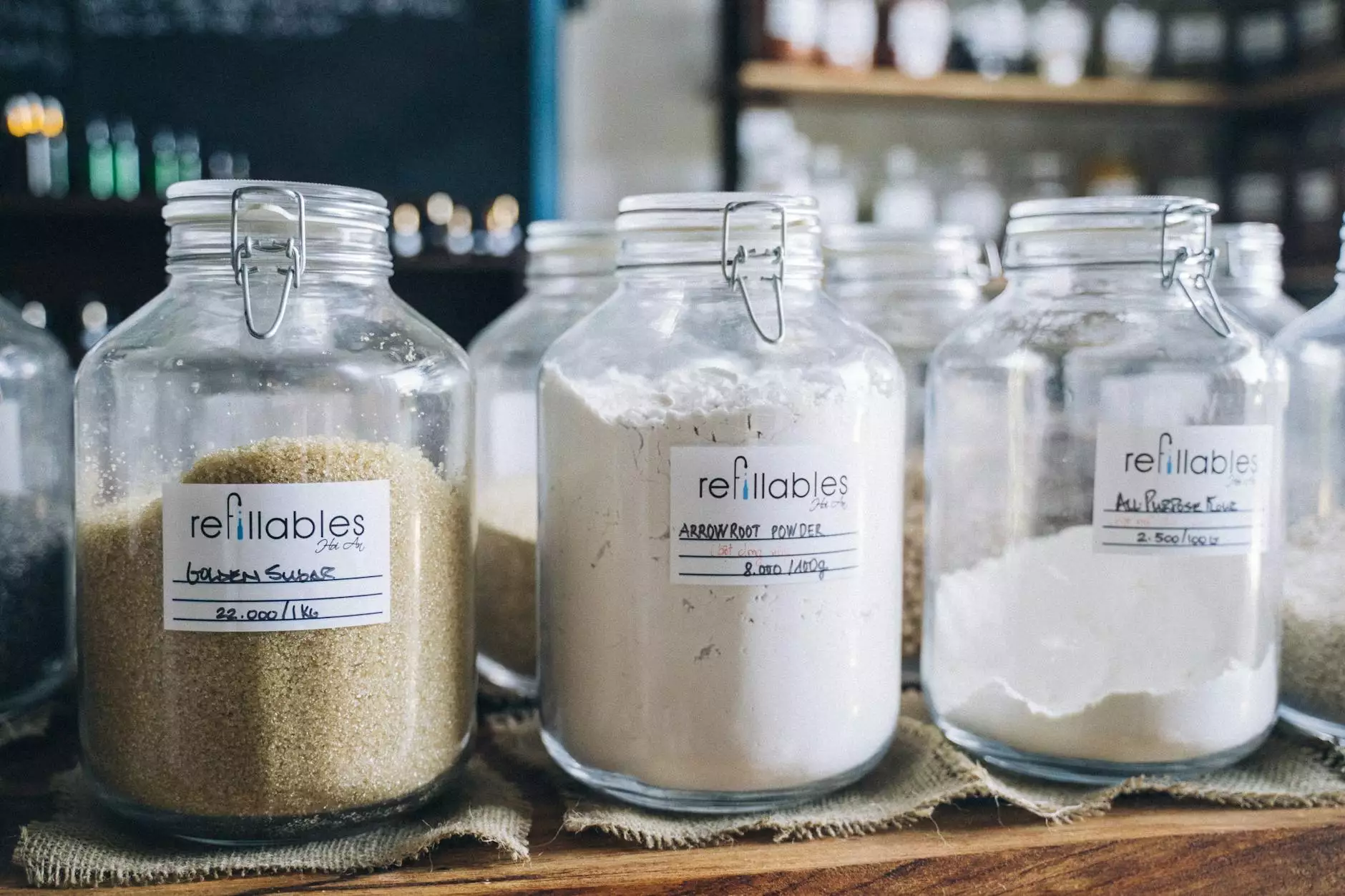
In today's digital age, data labeling has emerged as one of the foundational aspects of successful machine learning applications. As businesses strive to leverage cutting-edge technologies for better decision-making and improved customer experiences, understanding how data labeling operates becomes critically important. This article will delve into the intricacies of data labeling, its relevance to machine learning, and how it can significantly impact businesses, particularly in the sectors of Home Services and Keys & Locksmiths.
What is Data Labeling?
Data labeling is a crucial process in machine learning that involves annotating data points so that machines can understand and learn from them. This practice transforms raw data into a structured format, which can be utilized by algorithms to make predictions or decisions.
The Process of Data Labeling
Data labeling typically involves several key steps:
- Data Collection: Gathering the necessary raw data from various sources.
- Annotation: Adding labels or tags to the data to categorize it appropriately.
- Quality Assurance: Ensuring the accuracy of labels through verification and correction processes.
- Integration: Incorporating the labeled data into machine learning models.
Why Data Labeling is Essential for Machine Learning
Machine learning algorithms depend heavily on labeled data to learn patterns, make decisions, and predict outcomes. Here are several reasons why data labeling is essential:
1. Enhances Model Accuracy
When data is accurately labeled, machine learning models are able to learn from the data more effectively, leading to higher accuracy in predictions. This is particularly critical for businesses in the Keys & Locksmiths sector, where precision can determine the success or failure of a service.
2. Facilitates Effective Training
Quality training data allows models to generalize better, making them more effective in real-world applications. In Home Services, for example, models trained on well-labeled datasets can optimize service delivery based on customer preferences.
3. Reduces Bias
Uniformity in data labeling helps minimize bias in machine learning models. By ensuring diverse and representative data labeling, businesses can develop more equitable and just algorithms that serve all customer segments effectively.
The Role of Data Labeling in Home Services and Locksmithing
As we explore the implications of data labeling on specific industries, we can see that the Home Services and Keys & Locksmiths sectors stand to gain significantly from these practices. Here are some examples of how data labeling influences these domains:
1. Customer Service Optimization
In the Home Services industry, understanding customer needs is paramount. By analyzing labeled data from customer interactions, businesses can identify common queries and pain points, thus tailoring their services to enhance customer satisfaction.
2. Predictive Maintenance in Locksmithing
Data labeling can play a vital role in predictive maintenance for locksmith services. By labeling data on lock failures and repairs, machine learning models can predict when maintenance is needed, allowing locksmiths to offer proactive services, which can lead to increased customer loyalty and trust.
Challenges in Data Labeling
Despite its importance, data labeling comes with its own set of challenges that businesses must navigate:
1. Time-Consuming Process
The process of accurately labeling data can be labor-intensive and time-consuming, which can delay machine learning projects. However, investing time in high-quality data labeling pays off in the long run with improved model performance.
2. Expertise Required
Labeling data correctly often requires domain expertise, particularly in specialized fields such as locksmith services or home repairs. Incorrect labels can severely impact model accuracy, leading to flawed business insights.
3. Cost Implications
Depending on the volume and complexity of data, the costs associated with data labeling can escalate. Businesses must weigh these costs against the potential return on investment from improved machine learning outcomes.
Strategies for Effective Data Labeling
To overcome the challenges associated with data labeling, businesses can implement several strategies:
1. Leveraging Technology
Utilizing labeling tools and software can expedite the data labeling process, ensuring that it is both efficient and accurate. Advanced tools use artificial intelligence to aid in labeling tasks, significantly reducing the manual effort involved.
2. Hiring Skilled Professionals
Investing in skilled data annotators who possess the necessary domain knowledge ensures that the labeling process is conducted accurately. Whether it’s for Home Services or locksmith tasks, domain expertise translates into better quality data.
3. Implementing a Quality Assessment Process
Establishing a robust quality assurance process that reviews and verifies the labeled data can help in maintaining consistency and accuracy over time. Regular audits can help identify and correct errors in labeling.
Future Trends in Data Labeling for Machine Learning
As machine learning technology evolves, several trends are emerging in the field of data labeling that businesses should keep an eye on:
1. Automation of Data Labeling
With the rapid advancements in AI, automation is set to transform data labeling processes. Automated labeling tools are becoming increasingly sophisticated, enabling faster and more cost-effective data preparation.
2. Crowdsourcing for Data Labeling
Crowdsourced data labeling is gaining traction, providing businesses access to a larger pool of annotators who can label data quickly and at scale. This approach can be particularly beneficial for industries like locksmithing, where diverse data input is valuable.
3. Enhanced Collaboration Across Teams
Data labeling is becoming more collaborative, with cross-functional teams working together to ensure the data accurately reflects the needs and goals of the business. Enhanced communication can lead to more effective data labeling processes.
Conclusion: The Key to Business Success
In conclusion, the role of data labeling in machine learning cannot be overstated, especially in sectors like Home Services and Keys & Locksmiths. By investing the necessary time, resources, and expertise into effective data labeling practices, businesses can unlock the full potential of machine learning technologies and drive better outcomes for their customers.
As the landscape of machine learning continues to evolve, those who prioritize high-quality data labeling will be well-positioned to stay ahead of their competition and achieve sustained success in their respective industries.
data labeling machine learning